第 2 章 Vegan 使用技巧
R package for community ecologists: popular ordination methods, ecological null models & diversity analysis
Vegan 是面向研究群落的生态学家开发的软件包,包括了流行的坐标分析方法、生态学的零假设和多样性分析方法。
2.1 计算 beta-dispersion
使用 betadisper()
计算。这是一个 beta-diversity (β多样性)指标。
example("betadisper")
2.1.1 使用 varespec
数据集计算 beta-dispersion
2.1.1.1 数据集说明
varespec
数据有 24 行和 44 列,是 44 个物种(species)在 24 个样品(site)中的估计覆盖率,
列名是 44 个物种拉丁名称的简写。varechem
数据有 24 行、14 列,是 24 个样品
中 14 个土壤相关的属性。
2.1.1.2 样本间距
vegdist
默认计算样品间的 Bray-Curtis 距离,其定义为:
\[d_{jk} = (\sum abs(x_{ij}-x_{ik}))/(\sum (x_{ij}+x_{ik}))\]
其中,\(x_{ij}\) 和 \(x_{ik}\) 分别代表物种(列) \(i\) 在两个样品(行)\(j\) 和 \(k\) 中的数量。 则前两个位点的 Bray-Curtis 距离是 0.5310021。
2.1.1.3 样本分组及计算结果
## First 16 sites grazed, remaining 8 sites ungrazed
groups <- factor(c(rep(1,16), rep(2,8)), labels = c("grazed","ungrazed"))
## Calculate multivariate dispersions
mod <- betadisper(dis, groups)
mod
##
## Homogeneity of multivariate dispersions
##
## Call: betadisper(d = dis, group = groups)
##
## No. of Positive Eigenvalues: 15
## No. of Negative Eigenvalues: 8
##
## Average distance to median:
## grazed ungrazed
## 0.3926 0.2706
##
## Eigenvalues for PCoA axes:
## (Showing 8 of 23 eigenvalues)
## PCoA1 PCoA2 PCoA3 PCoA4 PCoA5 PCoA6 PCoA7 PCoA8
## 1.7552 1.1334 0.4429 0.3698 0.2454 0.1961 0.1751 0.1284
mod
返回一个 betadisper 类的列表,有以下几个元件:
eig
:numeric;PCoA 分析的特征值vectors
: matrix;PCoA 分析特征向量distances
: numeric;在 PCoA 的多维空间中,每个样本与其对应的分组中心间的欧氏距离。group
: factor;分组信息。centroids
: matrix;分组在 PCoA 坐标系中的中心点。call
: 函数调用信息。
str(mod)
## List of 7
## $ eig : Named num [1:23] 1.755 1.133 0.443 0.37 0.245 ...
## ..- attr(*, "names")= chr [1:23] "PCoA1" "PCoA2" "PCoA3" "PCoA4" ...
## $ vectors : num [1:24, 1:23] 0.0946 -0.3125 -0.3511 -0.3291 -0.1926 ...
## ..- attr(*, "dimnames")=List of 2
## .. ..$ : chr [1:24] "18" "15" "24" "27" ...
## .. ..$ : chr [1:23] "PCoA1" "PCoA2" "PCoA3" "PCoA4" ...
## $ distances : Named num [1:24] 0.331 0.245 0.409 0.406 0.271 ...
## ..- attr(*, "names")= chr [1:24] "18" "15" "24" "27" ...
## $ group : Factor w/ 2 levels "grazed","ungrazed": 1 1 1 1 1 1 1 1 1 1 ...
## $ centroids : num [1:2, 1:23] -0.1455 0.2786 0.0758 -0.2111 -0.0137 ...
## ..- attr(*, "dimnames")=List of 2
## .. ..$ : chr [1:2] "grazed" "ungrazed"
## .. ..$ : chr [1:23] "PCoA1" "PCoA2" "PCoA3" "PCoA4" ...
## $ group.distances: num [1:2(1d)] 0.393 0.271
## ..- attr(*, "dimnames")=List of 1
## .. ..$ : chr [1:2] "grazed" "ungrazed"
## $ call : language betadisper(d = dis, group = groups)
## - attr(*, "class")= chr "betadisper"
## - attr(*, "method")= chr "bray"
## - attr(*, "type")= chr "median"
## - attr(*, "bias.adjust")= logi FALSE
这 24 个样品来自两组处理,其中前 16 个为一组(grazed),后 8 个为另一组(ungrazed)。
betadisper()
计算了每个组样品距离中心点(“中位数”)距离的平均值。输出中还包括
23 个特征值(eigenvalues)。
2.1.1.4 统计分析
To test if one or more groups is more variable than the others, ANOVA of the distances to group centroids can be performed and parametric theory used to interpret the significance of F. An alternative is to use a permutation test.
permutest.betadisper
permutes model residuals to generate a permutation distribution of F under the Null hypothesis of no difference in dispersion between groups.
anova()
方法被用来比较各个分组间是否存在有变化程度更大的情况。permutest()
则为这一任务提供了另一种统计分析手段。
## Perform test
anova(mod)
## Analysis of Variance Table
##
## Response: Distances
## Df Sum Sq Mean Sq F value Pr(>F)
## Groups 1 0.07931 0.079306 4.6156 0.04295 *
## Residuals 22 0.37801 0.017182
## ---
## Signif. codes: 0 '***' 0.001 '**' 0.01 '*' 0.05 '.' 0.1 ' ' 1
anova()
和 permutest()
分别计算显著性。
## Permutation test for F
permutest(mod, pairwise = TRUE, permutations = 99)
##
## Permutation test for homogeneity of multivariate dispersions
## Permutation: free
## Number of permutations: 99
##
## Response: Distances
## Df Sum Sq Mean Sq F N.Perm Pr(>F)
## Groups 1 0.07931 0.079306 4.6156 99 0.04 *
## Residuals 22 0.37801 0.017182
## ---
## Signif. codes: 0 '***' 0.001 '**' 0.01 '*' 0.05 '.' 0.1 ' ' 1
##
## Pairwise comparisons:
## (Observed p-value below diagonal, permuted p-value above diagonal)
## grazed ungrazed
## grazed 0.04
## ungrazed 0.04295
TukeyHSD()
方法则计算出两两 group 之间存在的差异及其显著性。
## Tukey's Honest Significant Differences
(mod.HSD <- TukeyHSD(mod))
## Tukey multiple comparisons of means
## 95% family-wise confidence level
##
## Fit: aov(formula = distances ~ group, data = df)
##
## $group
## diff lwr upr p adj
## ungrazed-grazed -0.1219422 -0.2396552 -0.004229243 0.0429502
plot(mod.HSD)
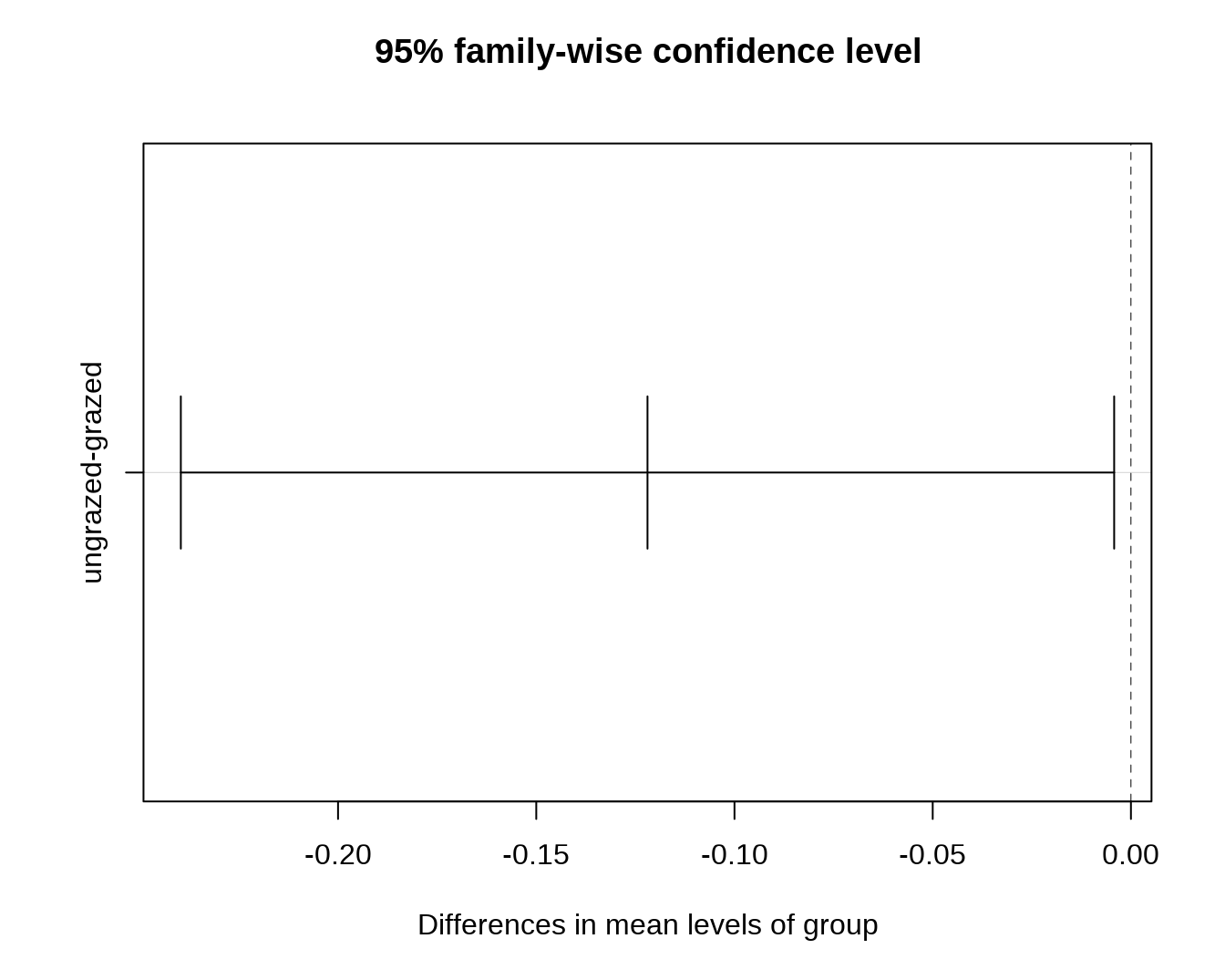
plot()
可以绘制样品和中心点之间的距离。
## Plot the groups and distances to centroids on the
## first two PCoA axes
plot(mod)
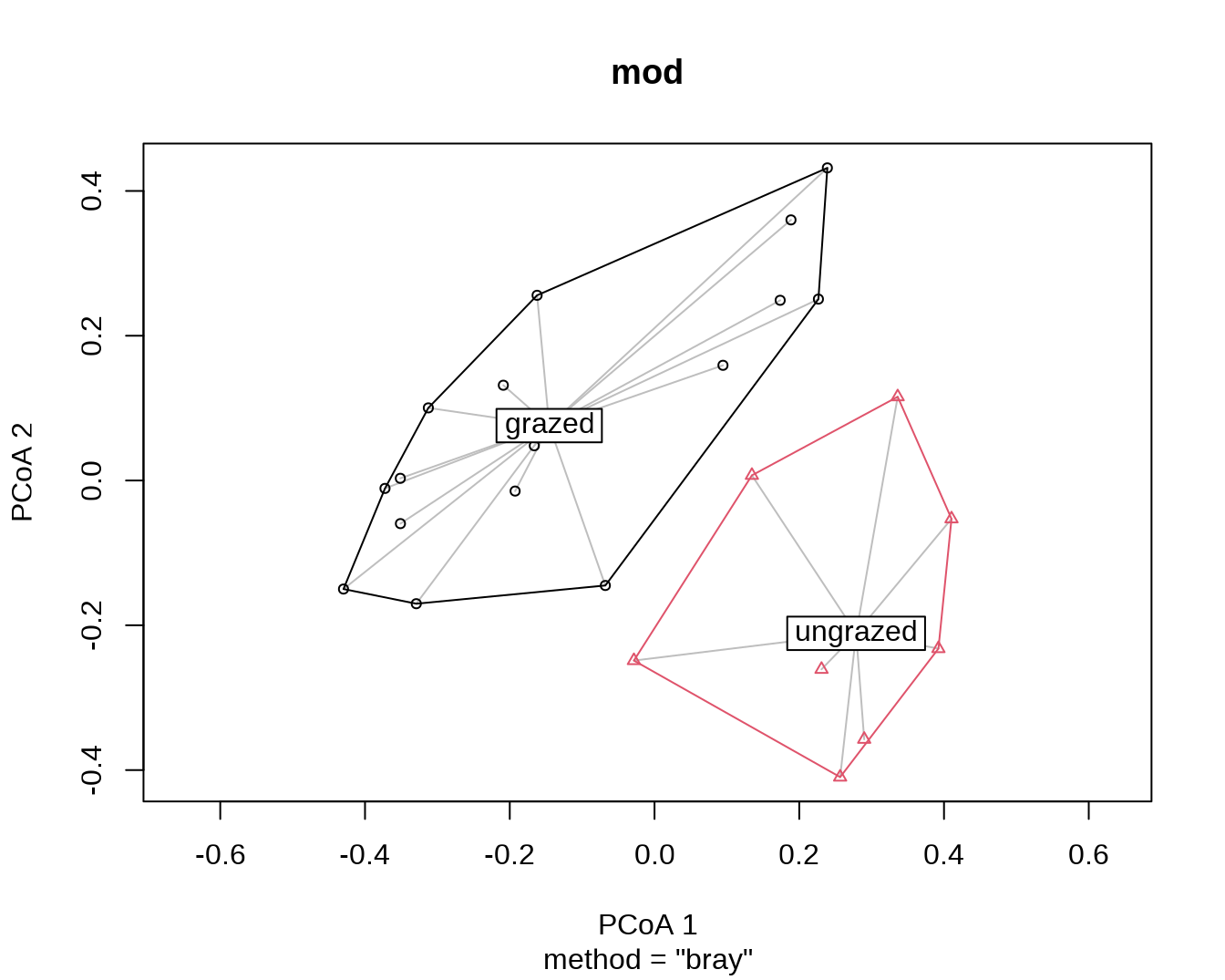
## with data ellipses instead of hulls
plot(mod, ellipse = TRUE, hull = FALSE) # 1 sd data ellipse
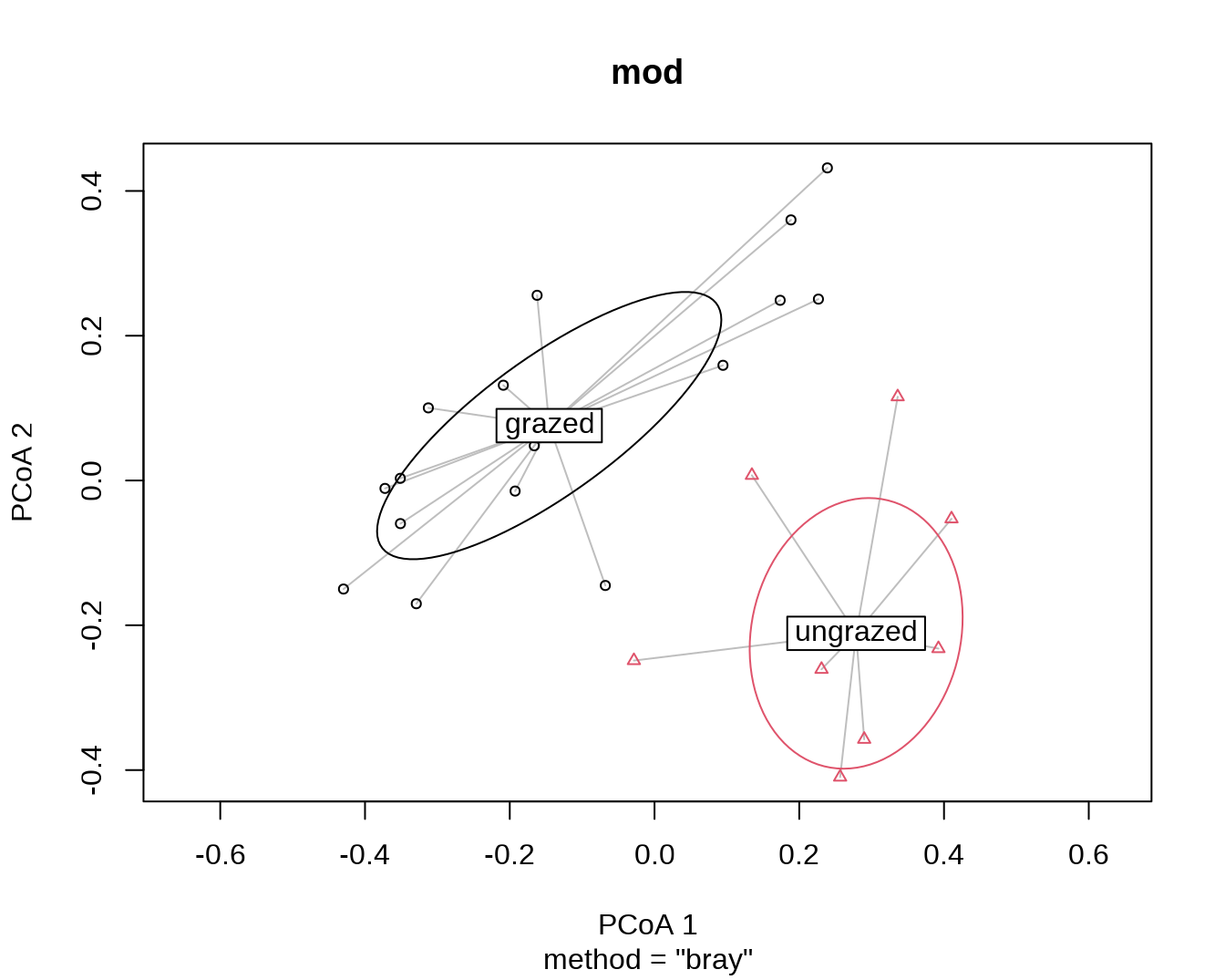
plot(mod, ellipse = TRUE, hull = FALSE, conf = 0.90) # 90% data ellipse
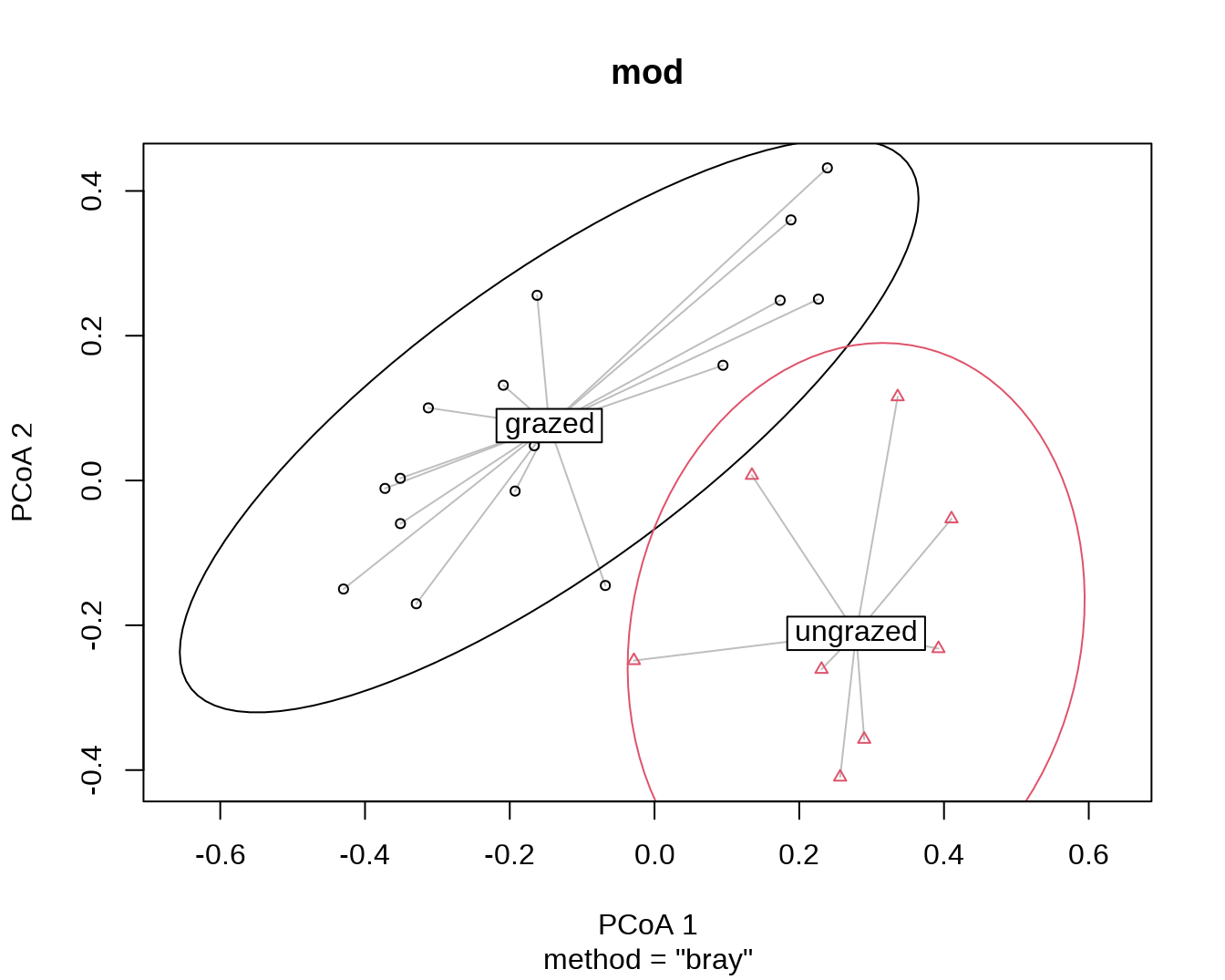
## can also specify which axes to plot, ordering respected
plot(mod, axes = c(3,1), seg.col = "forestgreen", seg.lty = "dashed")
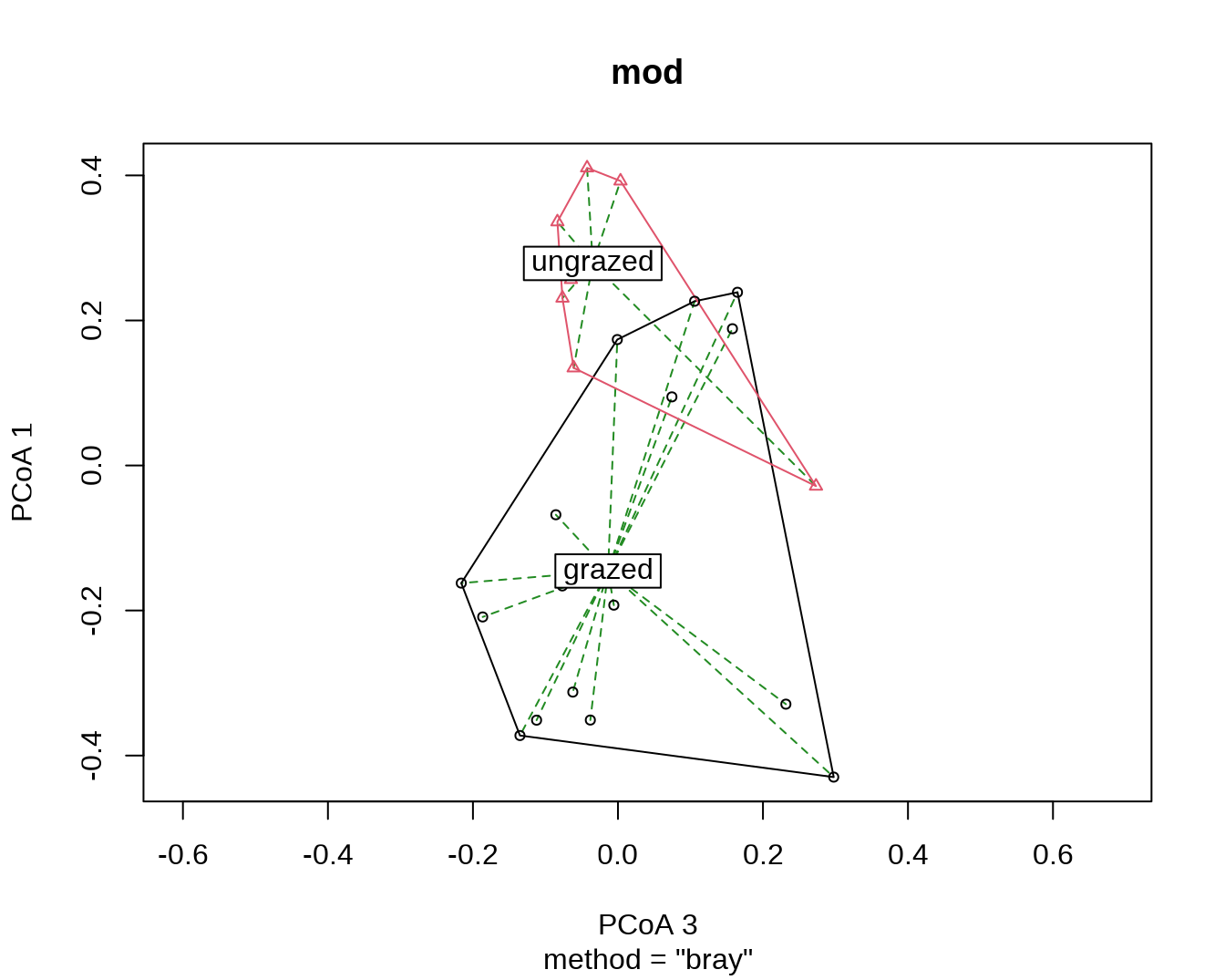
## Draw a boxplot of the distances to centroid for each group
boxplot(mod)
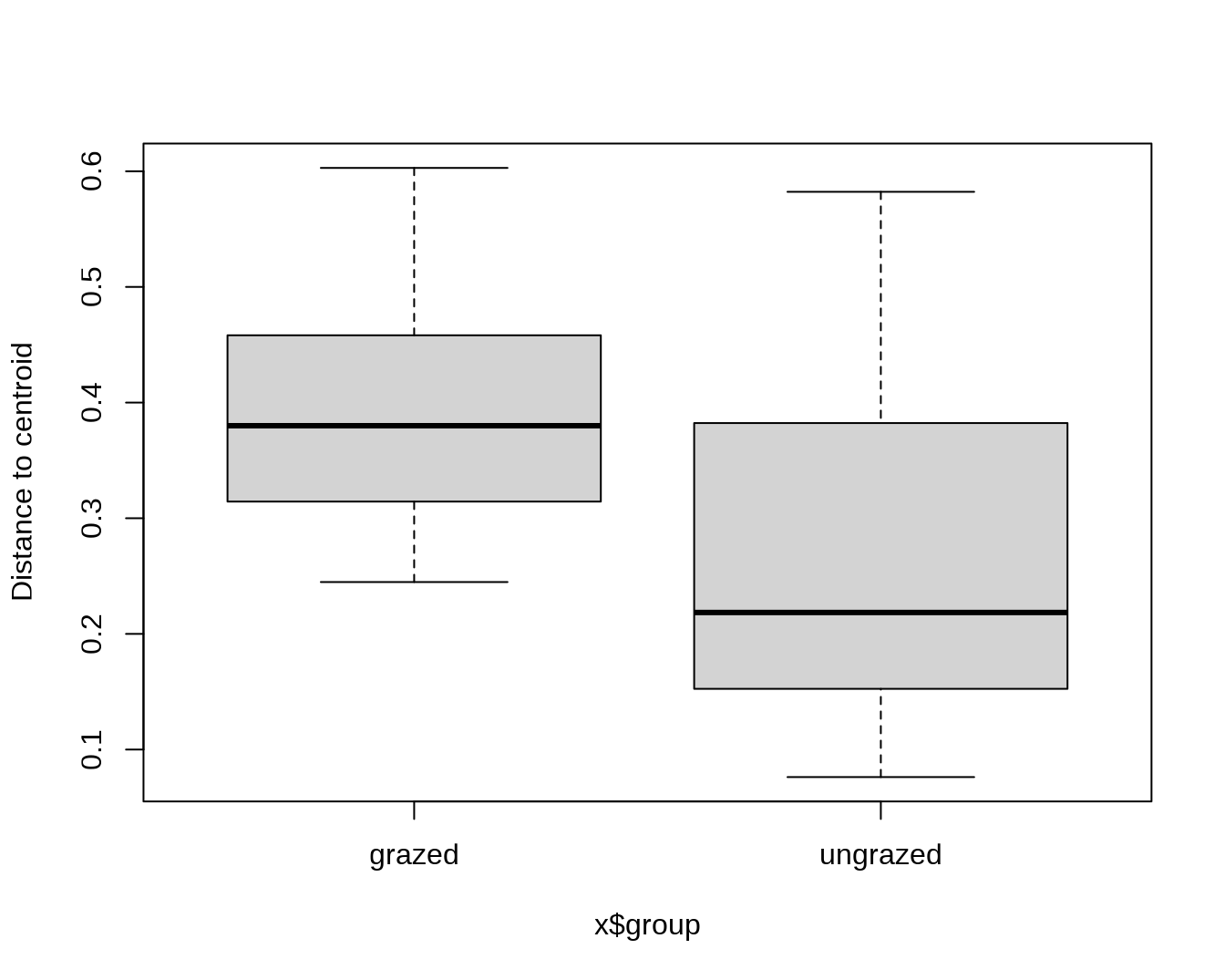
-
scores()
计算样本特征值。
## List of 2
## $ sites : num [1:24, 1:2] 0.0946 -0.3125 -0.3511 -0.3291 -0.1926 ...
## ..- attr(*, "dimnames")=List of 2
## .. ..$ : chr [1:24] "18" "15" "24" "27" ...
## .. ..$ : chr [1:2] "PCoA1" "PCoA2"
## $ centroids: num [1:2, 1:2] -0.1455 0.2786 0.0758 -0.2111
## ..- attr(*, "dimnames")=List of 2
## .. ..$ : chr [1:2] "grazed" "ungrazed"
## .. ..$ : chr [1:2] "PCoA1" "PCoA2"
# 可以将其画在图上
plot(scrs$sites, xlim = c(-.8, .8), ylim = c(-.5, .5))
text(scrs$sites, labels = rownames(scrs$sites), pos = 4)
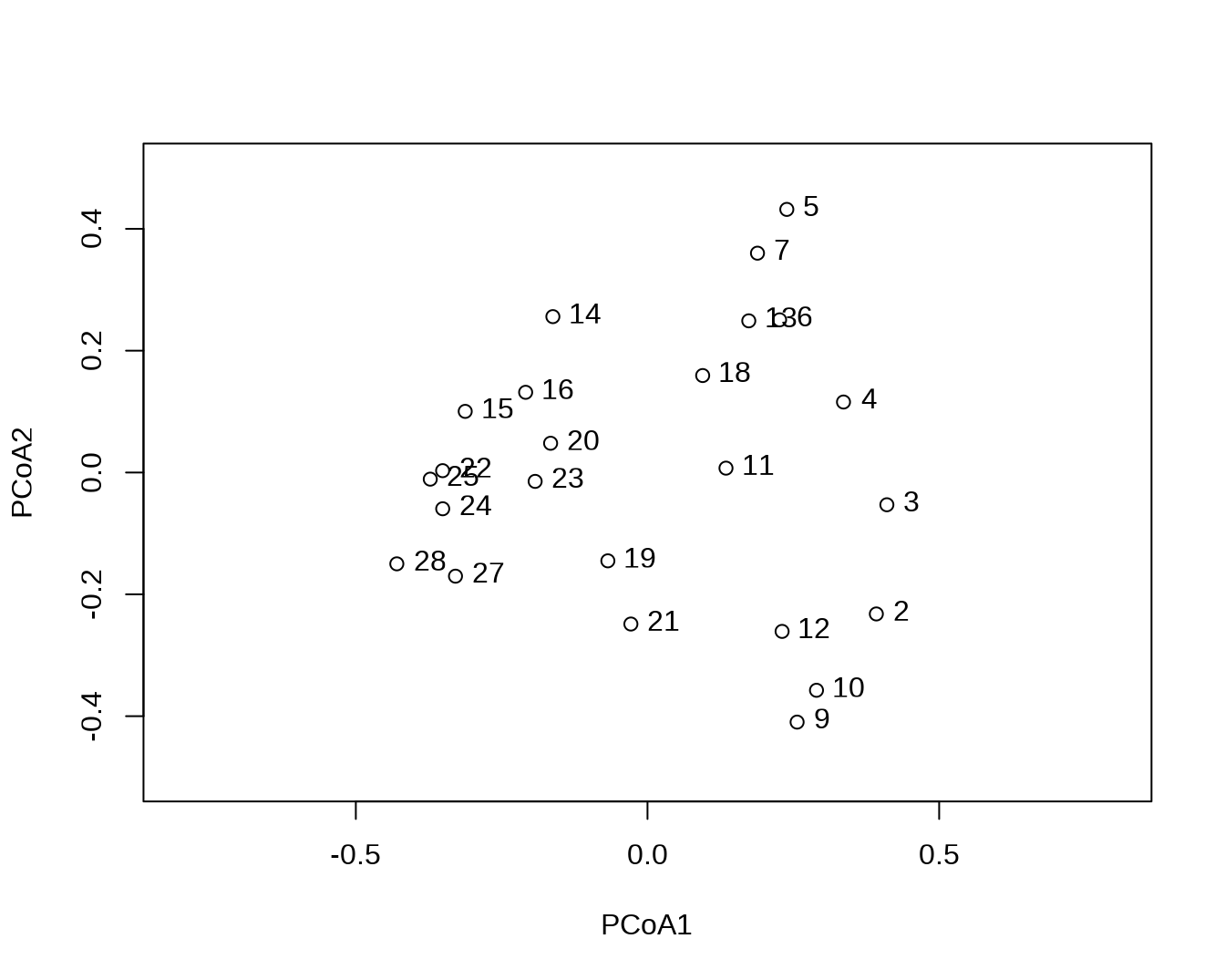
- 计算给出的样本、样本组的中心点坐标等。
## PCoA1 PCoA2 PCoA3 PCoA4
## 18 0.09459373 0.15914576 0.074400844 -0.202466025
## 15 -0.31248809 0.10032751 -0.062243360 0.110844864
## 24 -0.35106507 -0.05954096 -0.038079447 0.095060928
## 27 -0.32914546 -0.17019348 0.231623720 0.019110623
## 23 -0.19259443 -0.01459250 -0.005679372 -0.209718312
## 19 -0.06794575 -0.14501690 -0.085645653 0.002431355
# group centroids/medians
scores(mod, 1:4, display = "centroids")
## PCoA1 PCoA2 PCoA3 PCoA4
## grazed -0.1455200 0.07584572 -0.01366220 -0.0178990
## ungrazed 0.2786095 -0.21114993 -0.03475586 0.0220129
# eigenvalues from the underlying principal coordinates analysis
eigenvals(mod)
## PCoA1 PCoA2 PCoA3 PCoA4 PCoA5 PCoA6 PCoA7
## 1.7552165 1.1334455 0.4429018 0.3698054 0.2453532 0.1960921 0.1751131
## PCoA8 PCoA9 PCoA10 PCoA11 PCoA12 PCoA13 PCoA14
## 0.1284467 0.0971594 0.0759601 0.0637178 0.0583225 0.0394934 0.0172699
## PCoA15 PCoA16 PCoA17 PCoA18 PCoA19 PCoA20 PCoA21
## 0.0051011 -0.0004131 -0.0064654 -0.0133147 -0.0253944 -0.0375105 -0.0480069
## PCoA22 PCoA23
## -0.0537146 -0.0741390
-
bias.adjust = TRUE
对于 beta-多样性估计中的小样本偏差进行校正。
## try out bias correction; compare with mod3
(mod3B <- betadisper(dis, groups, type = "median", bias.adjust=TRUE))
##
## Homogeneity of multivariate dispersions
##
## Call: betadisper(d = dis, group = groups, type = "median", bias.adjust
## = TRUE)
##
## No. of Positive Eigenvalues: 15
## No. of Negative Eigenvalues: 8
##
## Average distance to median:
## grazed ungrazed
## 0.4055 0.2893
##
## Eigenvalues for PCoA axes:
## (Showing 8 of 23 eigenvalues)
## PCoA1 PCoA2 PCoA3 PCoA4 PCoA5 PCoA6 PCoA7 PCoA8
## 1.7552 1.1334 0.4429 0.3698 0.2454 0.1961 0.1751 0.1284
anova(mod3B)
## Analysis of Variance Table
##
## Response: Distances
## Df Sum Sq Mean Sq F value Pr(>F)
## Groups 1 0.07193 0.071927 3.7826 0.06468 .
## Residuals 22 0.41834 0.019015
## ---
## Signif. codes: 0 '***' 0.001 '**' 0.01 '*' 0.05 '.' 0.1 ' ' 1
permutest(mod3B, permutations = 99)
##
## Permutation test for homogeneity of multivariate dispersions
## Permutation: free
## Number of permutations: 99
##
## Response: Distances
## Df Sum Sq Mean Sq F N.Perm Pr(>F)
## Groups 1 0.07193 0.071927 3.7826 99 0.08 .
## Residuals 22 0.41834 0.019015
## ---
## Signif. codes: 0 '***' 0.001 '**' 0.01 '*' 0.05 '.' 0.1 ' ' 1
- 哪怕只有一个分组,仍然可以使用
## should always work for a single group
group <- factor(rep("grazed", NROW(varespec)))
(tmp <- betadisper(dis, group, type = "median"))
##
## Homogeneity of multivariate dispersions
##
## Call: betadisper(d = dis, group = group, type = "median")
##
## No. of Positive Eigenvalues: 15
## No. of Negative Eigenvalues: 8
##
## Average distance to median:
## grazed
## 0.4255
##
## Eigenvalues for PCoA axes:
## (Showing 8 of 23 eigenvalues)
## PCoA1 PCoA2 PCoA3 PCoA4 PCoA5 PCoA6 PCoA7 PCoA8
## 1.7552 1.1334 0.4429 0.3698 0.2454 0.1961 0.1751 0.1284
(tmp <- betadisper(dis, group, type = "centroid"))
##
## Homogeneity of multivariate dispersions
##
## Call: betadisper(d = dis, group = group, type = "centroid")
##
## No. of Positive Eigenvalues: 15
## No. of Negative Eigenvalues: 8
##
## Average distance to centroid:
## grazed
## 0.4261
##
## Eigenvalues for PCoA axes:
## (Showing 8 of 23 eigenvalues)
## PCoA1 PCoA2 PCoA3 PCoA4 PCoA5 PCoA6 PCoA7 PCoA8
## 1.7552 1.1334 0.4429 0.3698 0.2454 0.1961 0.1751 0.1284
- 当有缺失值的时候,也可以使用
## simulate missing values in 'd' and 'group'
## using spatial medians
groups[c(2,20)] <- NA
dis[c(2, 20)] <- NA
mod2 <- betadisper(dis, groups) ## messages
mod2
##
## Homogeneity of multivariate dispersions
##
## Call: betadisper(d = dis, group = groups)
##
## No. of Positive Eigenvalues: 14
## No. of Negative Eigenvalues: 5
##
## Average distance to median:
## grazed ungrazed
## 0.3984 0.3008
##
## Eigenvalues for PCoA axes:
## (Showing 8 of 19 eigenvalues)
## PCoA1 PCoA2 PCoA3 PCoA4 PCoA5 PCoA6 PCoA7 PCoA8
## 1.4755 0.8245 0.4218 0.3456 0.2159 0.1688 0.1150 0.1060
permutest(mod2, permutations = 99)
##
## Permutation test for homogeneity of multivariate dispersions
## Permutation: free
## Number of permutations: 99
##
## Response: Distances
## Df Sum Sq Mean Sq F N.Perm Pr(>F)
## Groups 1 0.039979 0.039979 2.4237 99 0.11
## Residuals 18 0.296910 0.016495
anova(mod2)
## Analysis of Variance Table
##
## Response: Distances
## Df Sum Sq Mean Sq F value Pr(>F)
## Groups 1 0.039979 0.039979 2.4237 0.1369
## Residuals 18 0.296910 0.016495
plot(mod2)
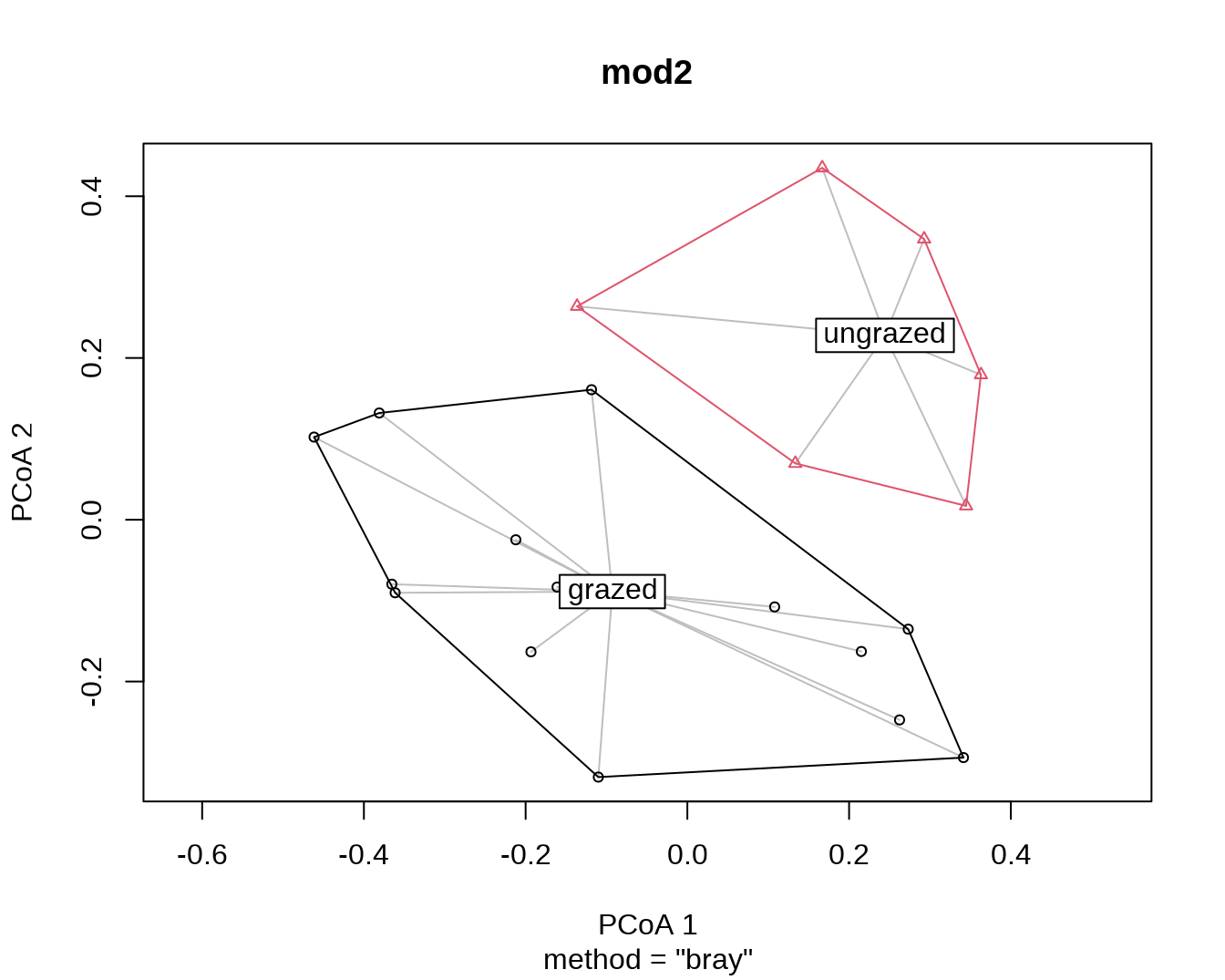
boxplot(mod2)
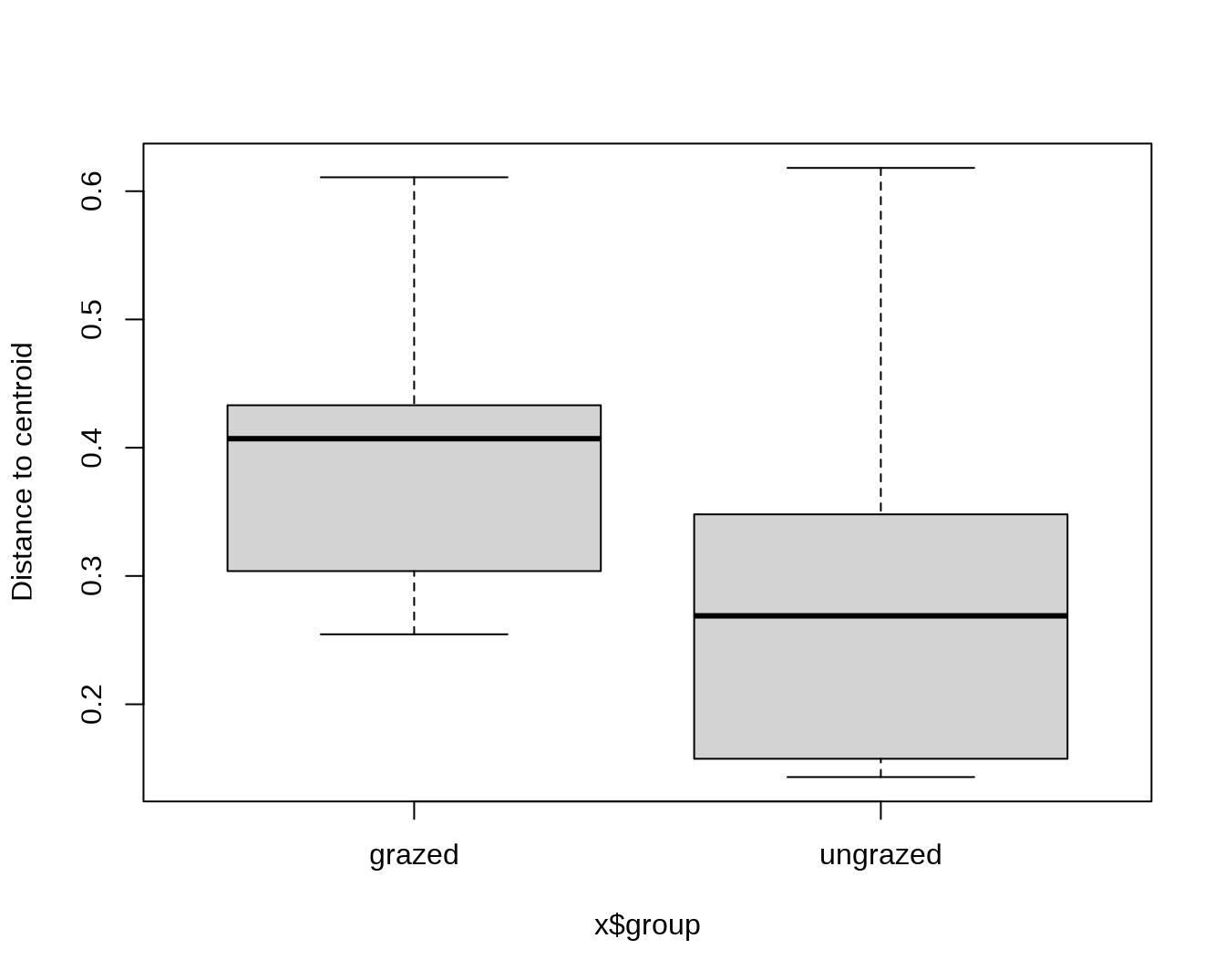
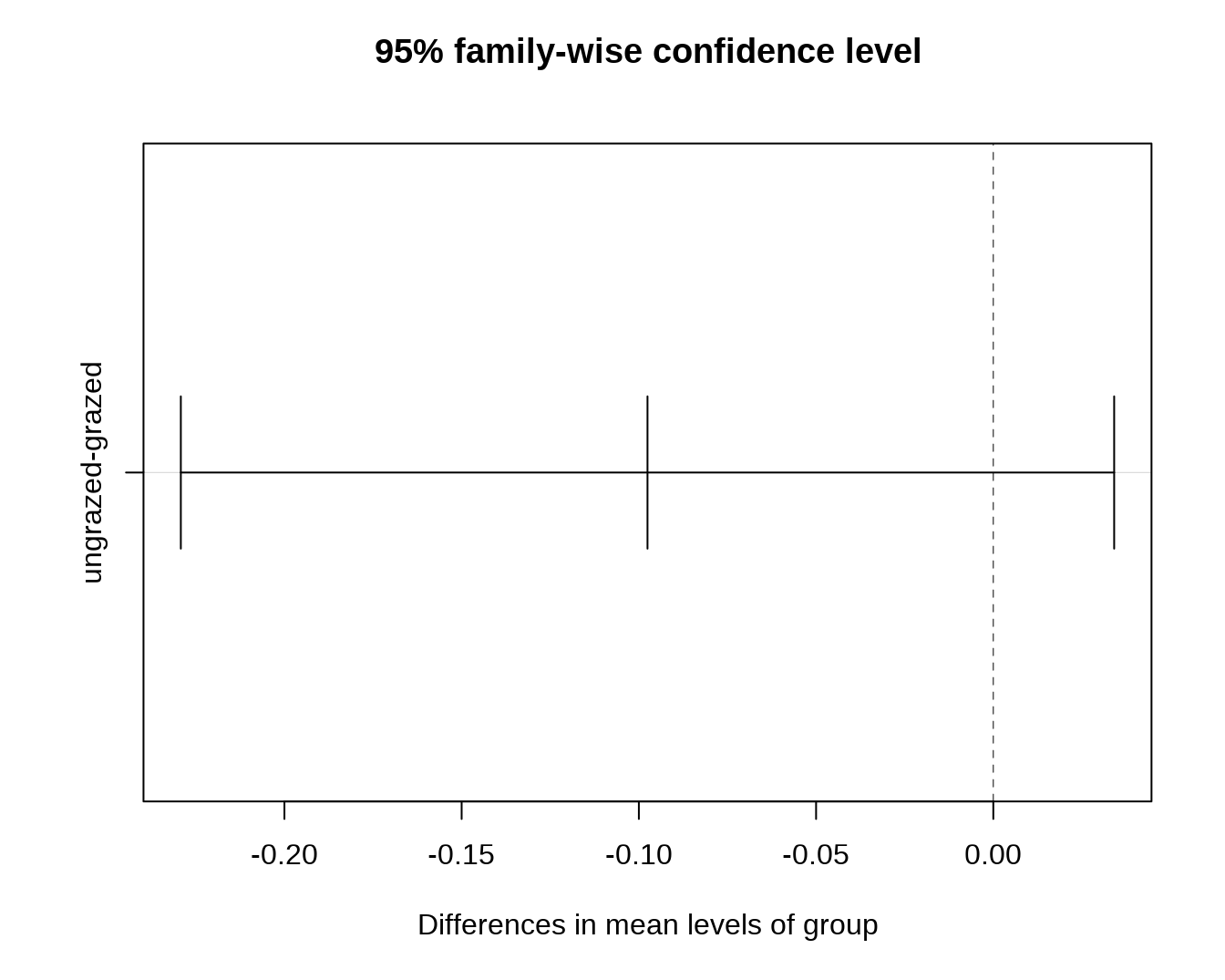
-
type = "centroid"
时的结果(默认为type = "median"
,一般不需要修改)。
## Using group centroids
mod3 <- betadisper(dis, groups, type = "centroid")
mod3
##
## Homogeneity of multivariate dispersions
##
## Call: betadisper(d = dis, group = groups, type = "centroid")
##
## No. of Positive Eigenvalues: 14
## No. of Negative Eigenvalues: 5
##
## Average distance to centroid:
## grazed ungrazed
## 0.4001 0.3108
##
## Eigenvalues for PCoA axes:
## (Showing 8 of 19 eigenvalues)
## PCoA1 PCoA2 PCoA3 PCoA4 PCoA5 PCoA6 PCoA7 PCoA8
## 1.4755 0.8245 0.4218 0.3456 0.2159 0.1688 0.1150 0.1060
permutest(mod3, permutations = 99)
##
## Permutation test for homogeneity of multivariate dispersions
## Permutation: free
## Number of permutations: 99
##
## Response: Distances
## Df Sum Sq Mean Sq F N.Perm Pr(>F)
## Groups 1 0.033468 0.033468 3.1749 99 0.1
## Residuals 18 0.189749 0.010542
anova(mod3)
## Analysis of Variance Table
##
## Response: Distances
## Df Sum Sq Mean Sq F value Pr(>F)
## Groups 1 0.033468 0.033468 3.1749 0.09166 .
## Residuals 18 0.189749 0.010542
## ---
## Signif. codes: 0 '***' 0.001 '**' 0.01 '*' 0.05 '.' 0.1 ' ' 1
plot(mod3)
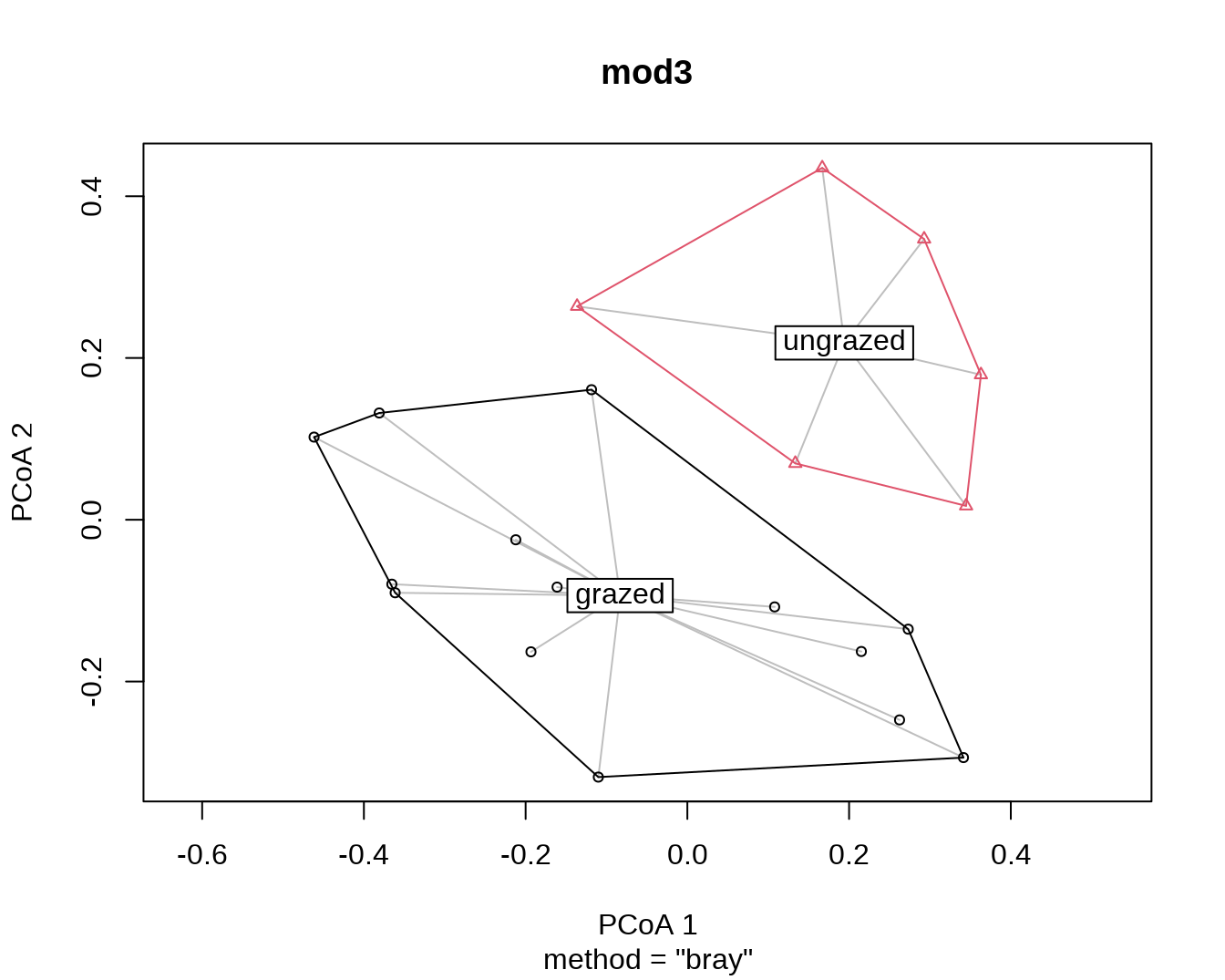
boxplot(mod3)
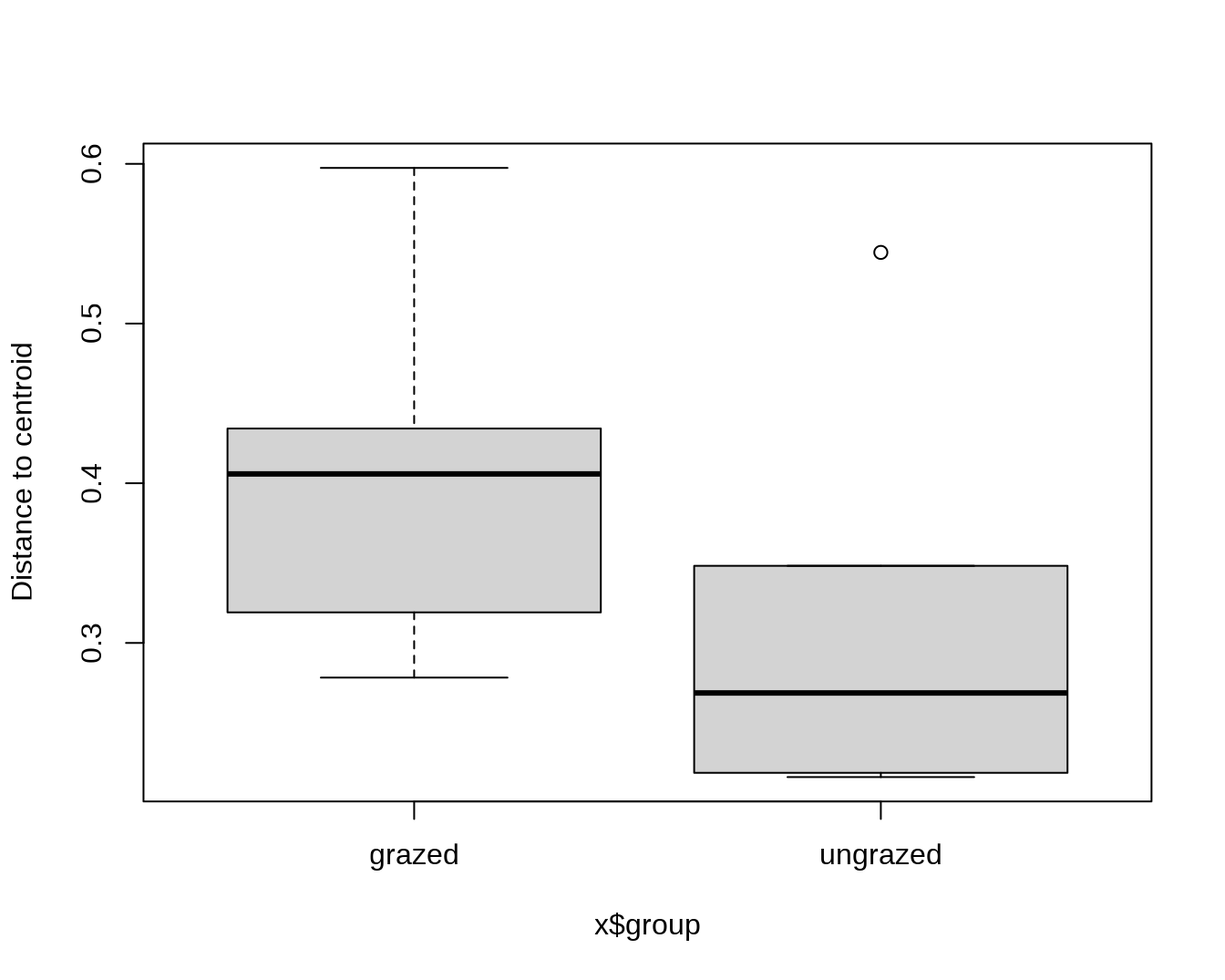
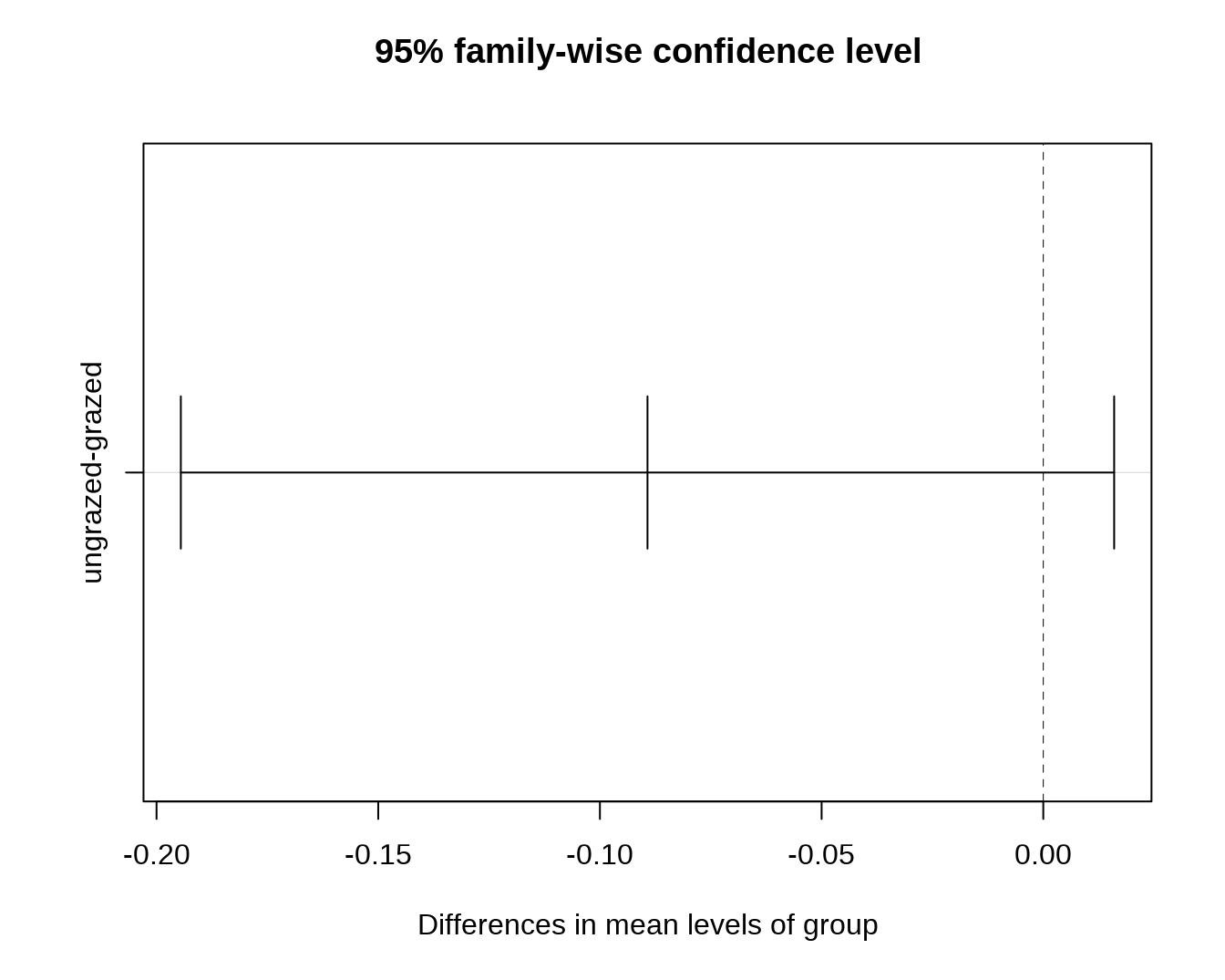
2.1.2 使用 dune
数据集计算 beta-dispersion
本例子来源于 Vignettes: Diversity analysis in vegan.
使用的数据是荷兰沙丘(dune)草地牧场(meadow)上的植被(vegetation)和环境因子。
- 使用
betadiver()
计算 beta-多样性。
betadiver()
的第二个参数指定计算 beta 多样性指标的算法。算法基于 \(a\), \(b\), \(c\) 等 3 个值(使用 betadiver(help = TRUE)
查看计算公式)。它们的含义如下:
method = "z"
时,计算的公式为:
\[z = (log(2) - log(2*a+b+c) + log(a+b+c))/log(2)\]
计算得到的 z
是一个 dist 对象。
z = betadiver(dune, method = "z")
z
## 1 2 3 4 5 6 7
## 2 0.4150375
## 8 9 10 11 12 13 14
## 2
## 15 16 17 18 19
## 2
## [ reached getOption("max.print") -- omitted 18 rows ]
按照 dune.env$Management
中给出的土地管理类型进行分组,计算 beta-dispersion。
(mod = with(dune.env, betadisper(z, Management)))
##
## Homogeneity of multivariate dispersions
##
## Call: betadisper(d = z, group = Management)
##
## No. of Positive Eigenvalues: 12
## No. of Negative Eigenvalues: 7
##
## Average distance to median:
## BF HF NM SF
## 0.2532 0.2512 0.4406 0.3635
##
## Eigenvalues for PCoA axes:
## (Showing 8 of 19 eigenvalues)
## PCoA1 PCoA2 PCoA3 PCoA4 PCoA5 PCoA6 PCoA7 PCoA8
## 1.65466 0.88696 0.53336 0.37435 0.28725 0.22445 0.16128 0.08099
对计算得到的结果进行可视化。
plot(mod)
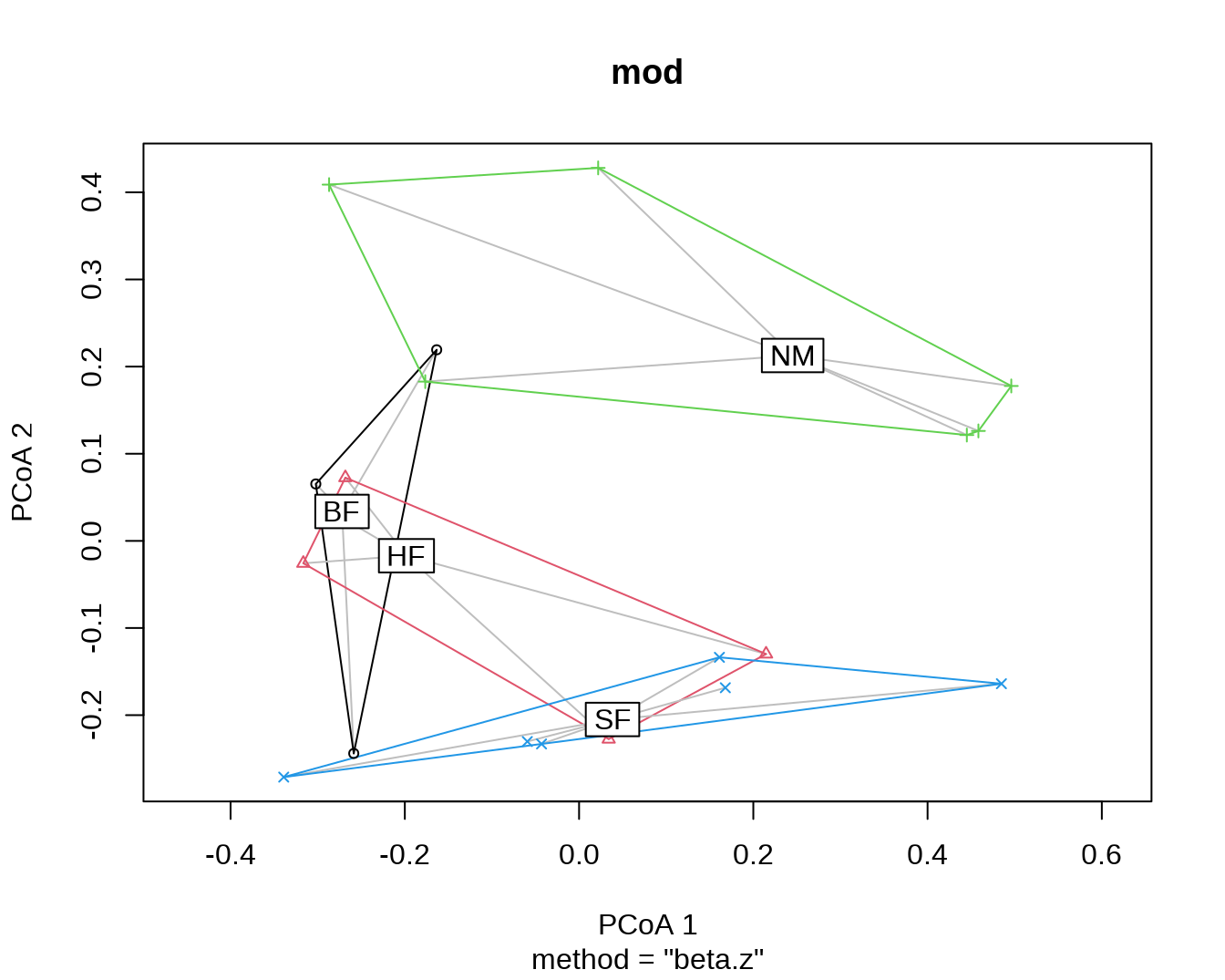
boxplot(mod)
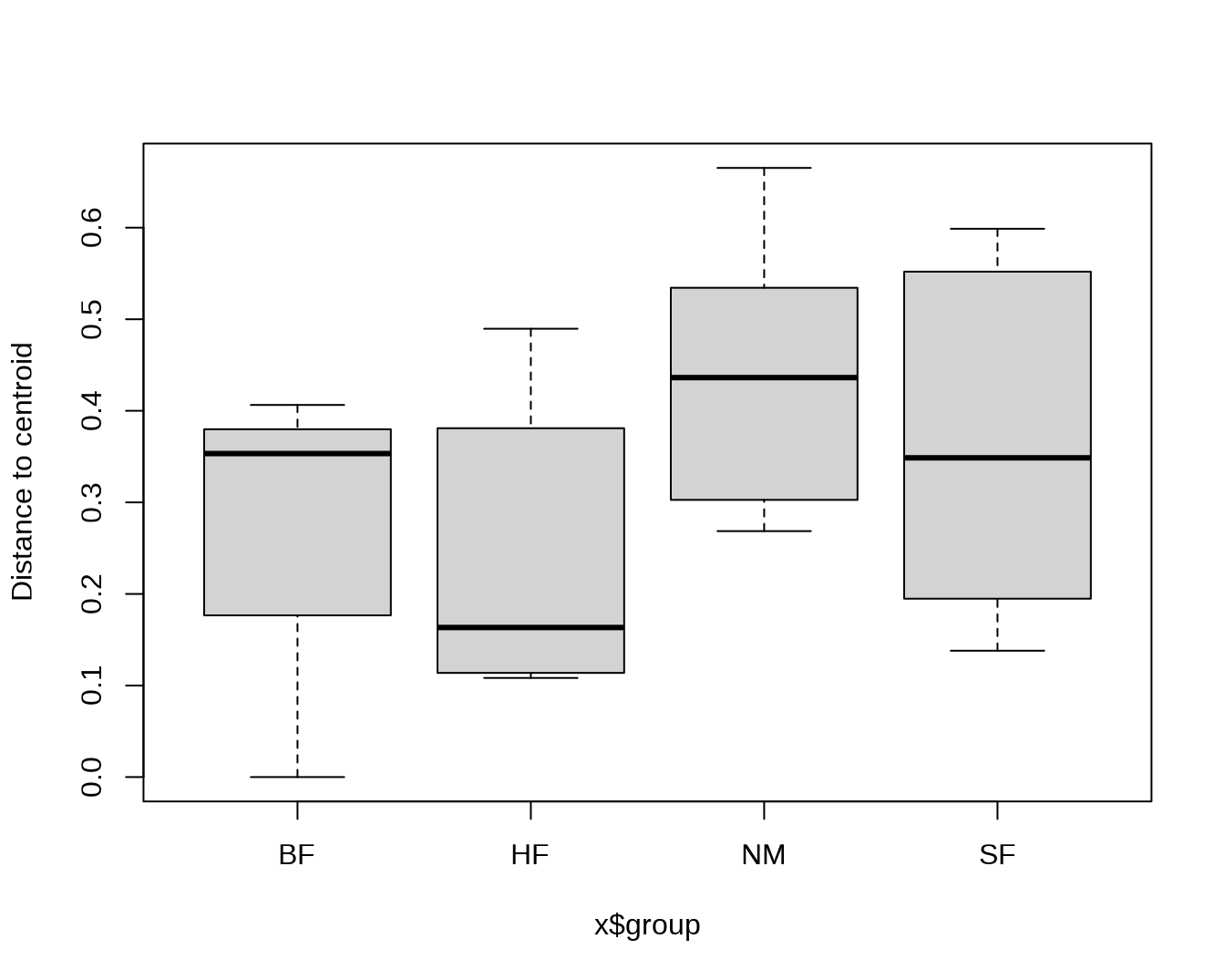